Deep learning for defected trees detection
farmB R&D team in cooperation with IBO’s Scientists prototyped a novel AI approach for the detection of trees with disease-infected leaves taken advantage of the latest developments in deep learning and object detection. The first disease type to be tested was anthracnose. So far, anthracnose machine learning based detection was performed on single leaf level and on images taken in controlled environment in terms of light and background clearness. The innovative approach is based on an object detector, namely a state-of-the-art Single Shot Detector (SSD), that was trained in detecting anthracnose-infected leaves on feature-rich images of walnut trees’ canopies under variable open-air orchard environment conditions.
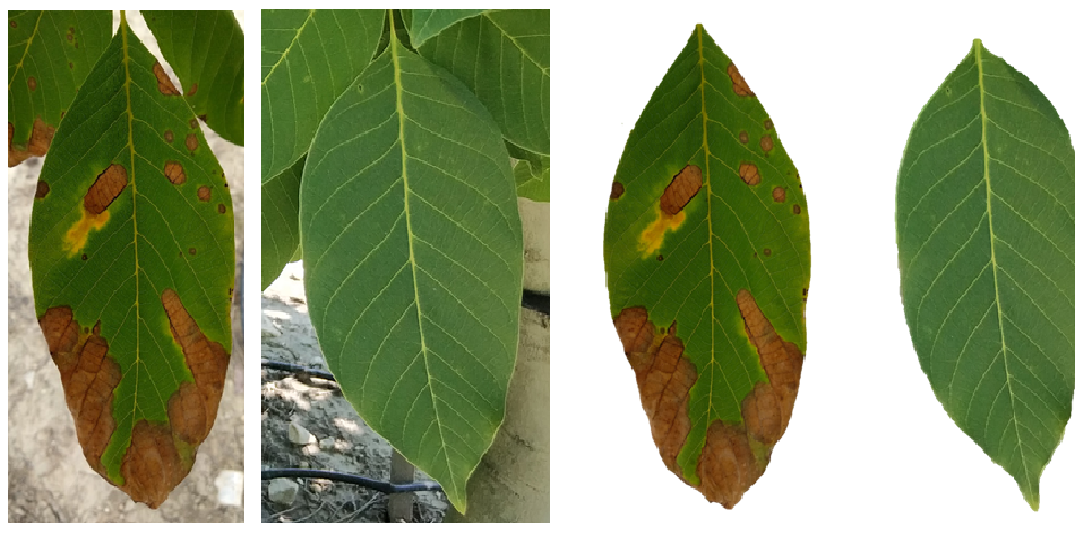
Focus of this study was to build an accurate and fast object detection system that can identify anthracnose-infected leaves on walnut trees, in order to be used in real agricultural environments. The encouraging results indicate that the detector shows great potential for direct application in commercial orchards, to detect infected leaves on tree level in real field conditions, and categorize the trees into infected or healthy in real time. Thus, this system can constitute an applicable solution for real-time scouting, monitoring, and decision making.
Disease detection of trees
A holistic approach for identifying disease-infected trees within orchards was developed. Based on novel AI algorithms, it is capable of classifying images of single infected leaves, as well as detecting them on tree-level images, under variable open-air orchard environment conditions.
State of the art deep learning algorithms such as convolutional neural networks (CNN) for classification tasks and a Single Shot Detector (SSD) for the object detection, are utilized to provide accuracy in its predictions and high-speed performance on deployment.
Primary use case of the approach is the identification of walnut trees infected with anthracnose. Focus of the study was to build a robust system, able to operate accurately within operational environments, aiming towards a system, directly applicable in commercial orchards.
The encouraging results demonstrated that such a system is not only possible, but it can produce predictions of high level of accuracy. Such a system can be used for scouting and monitoring, as well as for providing inputs to Decision Support Systems (DSS) on whether and where, fungicide application is necessary.
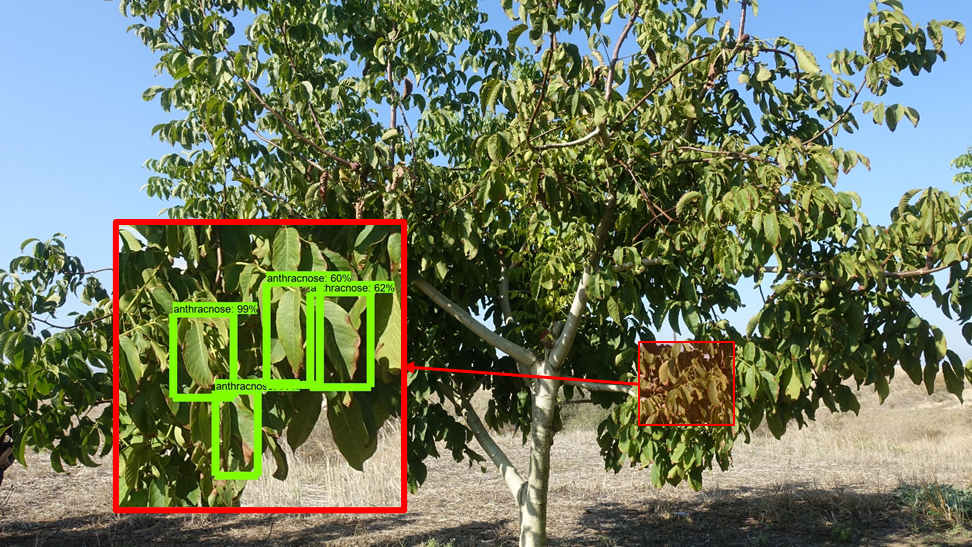